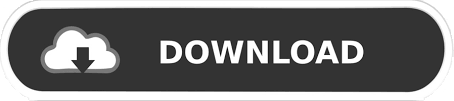
#SPSS 16 VARIABLE VIEW SCROLL BUG SOFTWARE#
Statistical tutorials and software guides.The ScrollView is a generic scrollable container, which scrolls multiple child components and views inside it. Retrieved Month, Day, Year, from įor example, if you viewed this guide on 29 th April 2020, you would use the following reference: Statistical tutorials and software guides. Introduction to robust estimation and hypothesis testing (3rd ed.). Chichester, West Sussex: John Wiley & Sons. Robust correlation: Theory and applications. Psychological transactions of the Royal Society of London A, 186, 343-414. Contributions to the mathematical theory of evolution. Glenview, IL: Scott, Foresman and Company. Introduction to bivariate and multivariate analysis. Introduction to mathematical statistics (7th. Effect of violation of normality on the t test of the correlation coefficient. Hoboken, NJ: John Wiley & Sons.Įdgell, S. Explaining psychological statistics (4th ed.). Statistical power analysis for the behavioral sciences (2nd ed.).
#SPSS 16 VARIABLE VIEW SCROLL BUG HOW TO#
To learn how to run a Pearson correlation in SPSS Statistics, go to our guide: Pearson's correlation in SPSS Statistics. You should write it as a proportion (e.g., r 2 = 0.36). However, you should not write r 2 = 36%, or any other percentage. It is sometimes expressed as a percentage (e.g., 36% instead of 0.36) when we discuss the proportion of variance explained by the correlation. It gives a measure of the amount of variation that can be explained by the model (the correlation is the model). The coefficient of determination, with respect to correlation, is the proportion of the variance that is shared by both variables. So, for example, a Pearson correlation coefficient of 0.6 would result in a coefficient of determination of 0.36, (i.e., r 2 = 0.6 x 0.6 = 0.36). The coefficient of determination, r 2, is the square of the Pearson correlation coefficient r (i.e., r 2). What is the Coefficient of Determination? By rejecting the null hypothesis, you accept the alternative hypothesis that states that there is a relationship, but with no information about the strength of the relationship or its importance. It simply tests the null hypothesis that there is no relationship. If your correlation coefficient has been determined to be statistically significant this does not mean that you have a strong association. You need to be careful how you interpret the statistical significance of a correlation. Yes, the easy way to do this is through a statistical programme, such as SPSS Statistics. Can I determine whether the association is statistically significant? If you have not tested the significance of the correlation then leave out the degrees of freedom and p-value such that you would simply report: r = -0.52. Where the degrees of freedom (df) is the number of data points minus 2 ( N – 2). You should express the result as follows: You need to state that you used the Pearson product-moment correlation and report the value of the correlation coefficient, r, as well as the degrees of freedom (df). How do I report the output of a Pearson product-moment correlation? As stated earlier, it does not even distinguish between independent and dependent variables. It can only establish the strength of linear association between two variables. No, the Pearson correlation cannot determine a cause-and-effect relationship. This is illustrated in the diagram below: This point is most easily illustrated by studying scatterplots of a linear relationship with an outlier included and after its removal, with respect to both the line of best fit and the correlation coefficient. Outliers can have a very large effect on the line of best fit and the Pearson correlation coefficient, which can lead to very different conclusions regarding your data. Why is testing for outliers so important? The diagram below indicates what a potential outlier might look like: This might be your best approach if you cannot justify removing the outlier. Alternatively, if you cannot justify removing the data point(s), you can run a nonparametric test such as Spearman's rank-order correlation or Kendall's Tau Correlation instead, which are much less sensitive to outliers. You can then either remove or manipulate that particular point as long as you can justify why you did so (there are far more robust methods for detecting outliers in regression analysis). You can detect outliers in a similar way to how you detect a linear relationship, by simply plotting the two variables against each other on a graph and visually inspecting the graph for wayward (extreme) points. Pearson Product-Moment Correlation (cont.) How can you detect outliers?Īn outlier (in correlation analysis) is a data point that does not fit the general trend of your data, but would appear to be a wayward (extreme) value and not what you would expect compared to the rest of your data points.
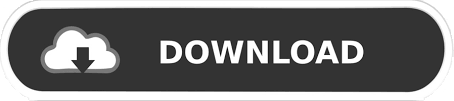